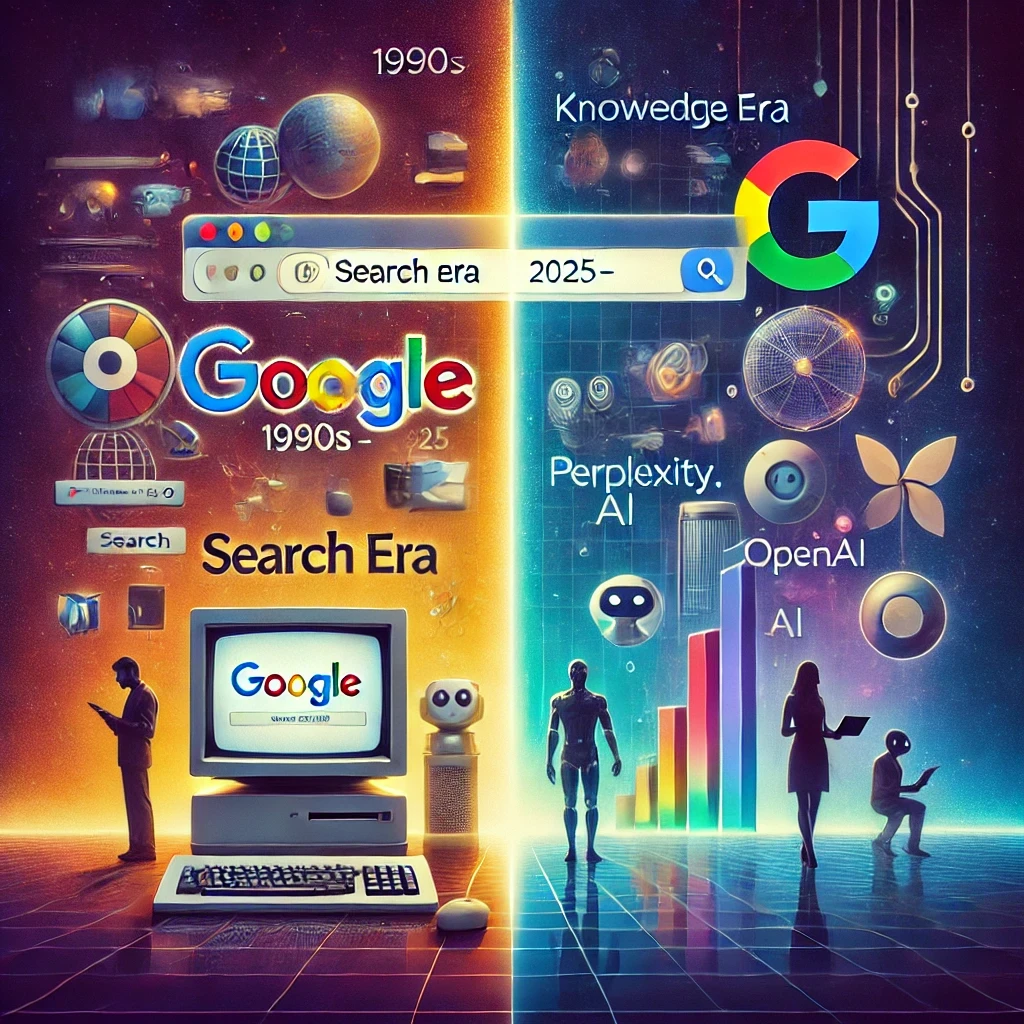
This article will explore the inevitable shift we are now seeing from an era that has been dominated by search, and the search engine, Google, to a new era that provides knowledge.
In the first era, which extended from the 1990s to the mid 2020s, we used search engines to gain knowledge. A user would go to a search engine, most often Google, key in their prompt – their keyword search query. The search engine would return several links, some paid links, and some organic links. This then required the user to click on one or several of those links, to start to answer their search query. This process was quite costly and inefficient for the user, in terms of time and effort to satisfy their query. But it also provided clicks, traffic, to the destination pages. This was the content publishers’ “quid pro quo” with the search engine: we provide great content, you provide valuable traffic. This balance resulted in a huge business for Google.
In the knowledge era, the user goes to a knowledge engine, and keys in their prompt, and the knowledge engine answers the prompt directly on its page. The searcher might then follow some links, if they are available, to verify what they have learned. This requires far less effort for the user, as the knowledge engine is doing all the heavy lifting. Verifying the information, in case of hallucinations, has become the additional task. But that said, there’s real concern that this paradigm will mean less organic and paid clicks to the original sources of the content. This shift to the knowledge era is much more useful to web users. But it presents challenges to marketers who have relied on search as an important organic and paid acquisition channel.
Let’s look at some history.
In 1998, Google was born, and changed the search landscape almost immediately with its simple interface and its PageRank algorithm. PageRank recognized the importance of site authority, in combination with page relevance and keyword relevance, to organize its search results. It meant bye bye to Alta Vista, et al, which relied solely on keyword exact matches.
Since the late 1990s, Google has thoroughly dominated the search era. It has the dominant web browser, collecting enormous amounts of data through the chrome browser. Google is also the dominant analytics platform, and Gmail is the dominant email provider. Google owns more than 90% of the mobile search traffic, and has been able to capture hundreds of billions of dollars in value, through their ad platforms, principally from their search platform.
Since the late 1990s, Google has kept innovating in the search space, with the singular goal of providing better search results, to improve the trust it has with its users. This moved search from simple keyword matching, to semantically-related keyword search results, to the emergence of zero position content on the results page.
In the 2012 Google began to provide direct answers which didn’t require a click, through its knowledge panel results for entity-specific searches, and in 2014 it introduced featured snippets. This was Google’s early move towards the knowledge era. But clicks to destination pages still happened, and Google managed the fine balance of providing a great user experience while also sending traffic to those who provide the content on the internet.
Google’s move into the use of artificial intelligence and machine learning, to continue to improve its search results, began with updates to its Hummingbird algorithm, and more formally in 2015 with the introduction of its RankBrain algorithm. It used artificial intelligence to better understand user intent – the real meaning behind a search query – rather than relying solely in keyword matching. It could do this with natural language processing (NLP) and pattern recognition analysis of the search queries. Google was now also able to learn from user behaviour, and adjust its search results accordingly.
Neural Networks, a detour.
Recurrent Neural Networks, RNNs, were the neural network architecture of this time, but they had their limitations. They relied on processing language sequentially, which reduced the capability for longer-term memory, and thus were not an effective solution for knowledge generation.
In the Spring of 2017, eight Googlers authored the paper, “Attention is All You Need” and a new Neural Net architecture was born – Transformers. This self-attention model could process a collection of words together, rather than only each word in a sequence, processing data in parallel, rather than sequentially. It was better able to handle long range dependencies in sentence structure. Attention, in the name of the title of the paper, refers to the ability to focus on particular parts of a sentence, in natural language processing. Transformers refers to the notion of transforming information.
The eight authors of the paper have subsequently left Google. One, Lukasz Kaiser, joined OpenAI. This transformer architecture laid the foundation for Google’s BERT algorithm.
In October, 2018, Google rolled out BERT (Bidirectional Encoder Representations from Transformers), a transformer-based language model, using the transformer architecture for language understanding. This helped Google better understand the nuances and context of language.
But then Google paused this direction of innovation. It clearly faced a significant challenge; Google was earning $146 billion in revenue from its search ads business in 2020, and the majority of that revenue came from its search business. Google needed to develop a similar business model for this emerging knowledge era. Google’s search era business model relies on clicks away from the search engine results page. The knowledge era is a threat to that model. Google had gone all in on its current pathway, despite inventing this new paradigm.
Clayton Christensen wrote the book, Innovators Dilemma. Was Google now becoming a new great case study for this seminal work from another era?
A second issue, no doubt, is the potential for hallucinations with this new architecture, and the issues this presents in terms of trust. Trust has been key to the success of Google’s search business. It is not clear that the transformer architecture can ever overcome the issues of hallucinations, as it is simply a prediction model, predicting the next token, rather than a reasoning model. But that’s for another article.
In November 2022, OpenAI rolled out ChatGPT. This AI interface interacts with OpenAI’s large language models, all based on the transformer architecture: GPT 3, 3.5, 4 and so on. GPT stands for Generative pre-trained transformer. To this date, the general public was unaware of transformer technology and its capabilities. It was like magic. You could put a prompt into the search box, and get your answer, without needing to navigate to links to seek out those answers. The tool was useful for much more than just search, but for search, it was a different experience.
The combination of hallucinations and the inability to provide links to verify the information that was created by the bot, caused issues with the adoption of this technology for search; but where there’s a problem, solutions develop in our innovation culture.
Nearly two years on, Perplexity.ai is one example of a knowledge engine which responds to prompts by first doing a search against the user’s prompt, and then running those results against a large language model. This allows perplexity.ai to create their novel responses, while also attributing these responses with the links discovered in the initial web search. A very clever work around. Similarly, OpenAI has now rolled out SearchGPT. Google has rolled out Gemini, but appears to be playing a little catch up, and has made a few missteps along the way.
In Summary
Search was a means to an end. We don’t want to search and click and explore. We simply want answers to our questions; we want new understanding and new knowledge. Search required a lot of human effort. Knowledge engines provide answers. We can then check those answers, via attributions in those answers, or by follow up searches. But it is a different paradigm, where the engine is doing the work and synthesizing the results into a digestible format. Technology has now allowed us to make this shift.
Will Google dominate this knowledge era, much as they’ve dominated the search era, only time will tell. It’s an open question. They did invent the technology that enables the knowledge era, but they stalled in its roll out.
Implications for brands.
It may be several years before this new knowledge era fully plays out. But as brand marketers, we need to think about two key acquisition channels that we currently rely upon: paid search and organic search. Do we want our content to influence the returns from the large language models. We know this is a potentially big copyright issue for news media outlets, authors, artists and others who rely on their intellectual property for their livelihoods. But for brands, we want to be discovered. So we need to be in the training data.
Unlike with regular search, this is not a question of updating your content, then alerting the search engine to crawl your site. Large language models are updated infrequently. It’s an entirely different and expensive process.
And even if your data is included in the training of the LLMs, how are you able to use this to drive business success. In the search era it was clear, being well represented in search yielded direct clicks to your site. Being well represented in answers in the knowledge era, this is less clear. Certainly, it should help with branding, if you gain brand mentions in the answers. If the answers are attributed, then the knowledge engine is making it easier for the user to then follow up directly with you.
But do the rules of SEO, as dictated by Google for the last 25 years, apply to this knowledge era? They are perhaps a great starting point. SEO, over those 25 years has focused more and more on simply the provision of great content, to answer specific questions. That should still apply to the knowledge era. That great content needs to be understandable, and be useful in answering whatever niche questions that are important to you, and your business, throughout the customer journey for your ideal customers. And the meta data of that content, should make it easier for the engines to understand.
So SEO should be a good starting point. The challenge is to make sure you are in the training data in the first place.
For PPC, these knowledge engines have to find a viable business model. The freemium subscription model is likely not going to persist, nor generate enough positive revenue to cover the significant expense of training these LLMs and engaging with them. So a PPC model may emerge, but this may lag the use of these knowledge engines, much like early search PPC lagged the adoption of search. What this may mean for marketers, social media becomes a more important channel in the medium term.
Social has also taken some of the search traffic away from traditional search, and certainly with a younger audience, social is where we spend significant amounts of our time. So ramping up a social media marketing strategy may be a good way to mitigate for the potential decline in PPC traffic in the short term.
Finally, what will the knowledge era really look like. Will it be centralized, as we have had for the search era, where one site, Google, archives the internet, and we search Google’s archive? Will Google, Perplexity, OpenAI or another player be able to take that position, and develop a viable business model that allows them to be the knowledge gatekeeper?
Or will it be fundamentally decentralized.
Each website may deploy its own knowledge app, that facilitates queries on that site’s domain of knowledge.
The possibilities are really interesting, the future is unclear and very exciting.